Author: Dr. Funmi Adeoye, Ph.D.
Title: AI Researcher
Institution: University of Lagos
Email: funmi.adeoye@unilag.edu.ng
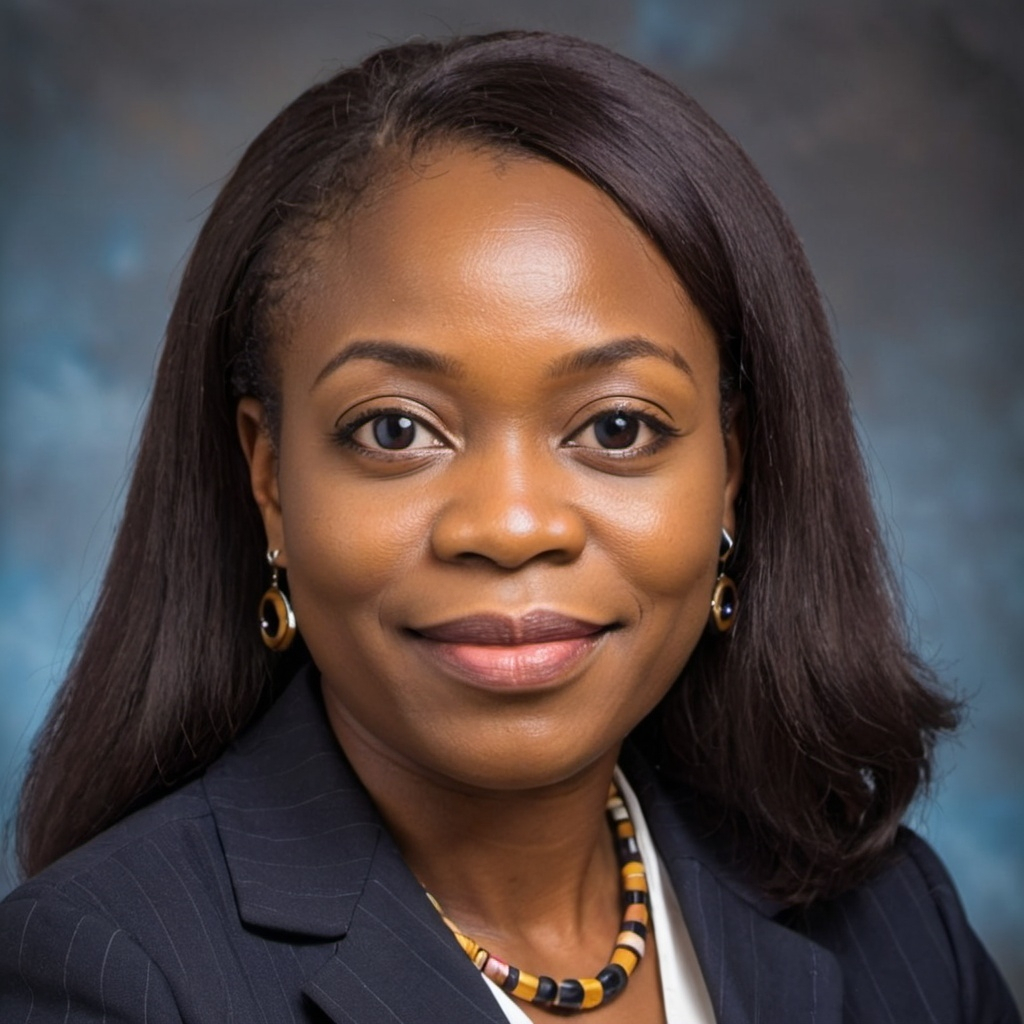
Abstract: Artificial intelligence (AI) is transforming healthcare diagnostics by enhancing accuracy, efficiency, and accessibility of medical diagnoses. This paper explores the applications, benefits, challenges, and future directions of AI in healthcare diagnostics, highlighting key technologies and case studies.
Introduction: AI technologies, including machine learning, deep learning, and natural language processing, are revolutionizing healthcare by providing advanced diagnostic tools. These technologies assist healthcare professionals in diagnosing diseases, predicting patient outcomes, and personalizing treatment plans.
Key Topics:
- AI Techniques in Diagnostics: Overview of AI techniques used in healthcare diagnostics, such as convolutional neural networks (CNNs) for image analysis, natural language processing (NLP) for medical record analysis, and machine learning algorithms for predictive analytics.
- Imaging and Radiology: Application of AI in medical imaging and radiology, including automated image analysis, detection of anomalies in X-rays, CT scans, MRIs, and ultrasound images. Examples of AI-driven diagnostic tools for detecting cancers, fractures, and neurological disorders.
- Pathology and Laboratory Medicine: Use of AI in pathology for analyzing histopathological images, identifying biomarkers, and automating lab processes. Impact on the accuracy and speed of diagnosing conditions such as cancers and infectious diseases.
- Predictive Analytics and Risk Assessment: Role of AI in predictive analytics for assessing patient risk factors, predicting disease progression, and identifying potential complications. Examples of AI models for predicting heart disease, diabetes, and patient readmission risks.
Applications and Benefits:
- Enhanced Diagnostic Accuracy: Improvement in diagnostic accuracy through AI’s ability to analyze large datasets, recognize patterns, and reduce human error.
- Efficiency and Speed: Acceleration of diagnostic processes, enabling faster decision-making and timely treatment interventions.
- Personalized Medicine: Facilitation of personalized treatment plans based on individual patient data, genetic information, and predicted responses to therapies.
Challenges and Considerations:
- Data Quality and Privacy: Ensuring high-quality, representative data for training AI models, and addressing privacy concerns related to patient data.
- Regulatory and Ethical Issues: Navigating regulatory frameworks and ethical considerations for AI in healthcare, including bias mitigation, transparency, and accountability.
- Integration with Clinical Workflows: Challenges in integrating AI tools with existing clinical workflows, ensuring usability, and gaining clinician trust and acceptance.
Future Directions: Future directions in AI for healthcare diagnostics include advancements in explainable AI for transparency, federated learning for data privacy, integration of multi-modal data for comprehensive diagnostics, and continuous learning systems to improve diagnostic accuracy over time.
Conclusion: AI is poised to significantly enhance healthcare diagnostics by providing powerful tools for early disease detection, accurate diagnosis, and personalized treatment. Addressing challenges and fostering collaboration between technologists, clinicians, and policymakers will be key to realizing the full potential of AI in healthcare.